AI risk management in finance: a necessary strategy
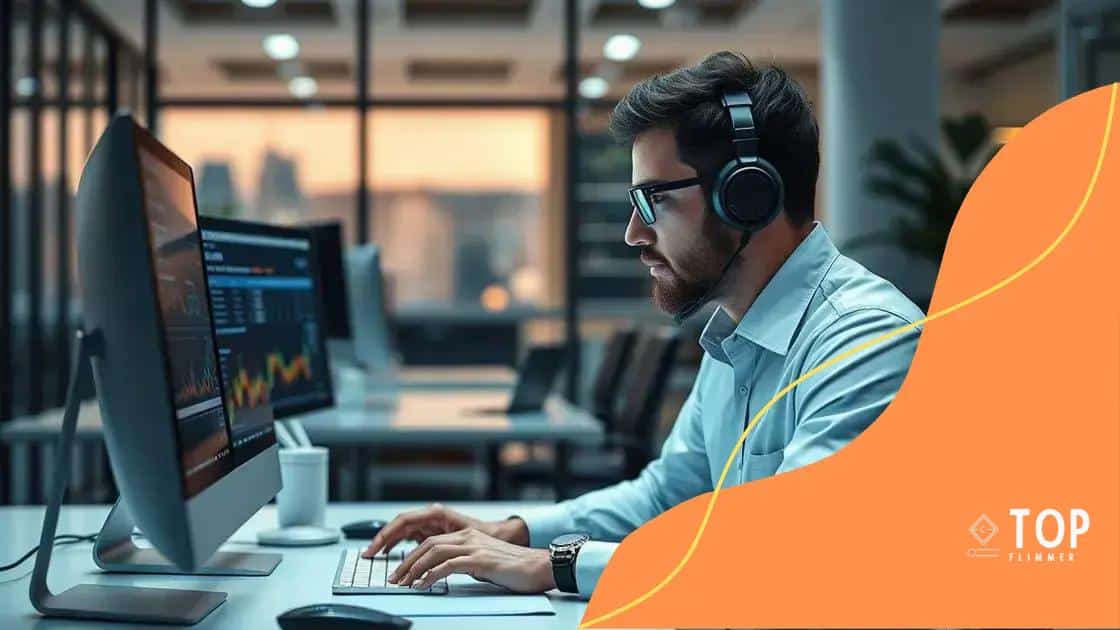
AI risk management in finance involves identifying and mitigating potential risks associated with AI technologies, including ethical concerns, regulatory compliance, data quality, and cybersecurity, to ensure safe implementation.
AI risk management in finance is becoming increasingly vital as organizations navigate the complexities of technological advancements. Have you ever wondered how financial institutions can balance innovation with security? This article will uncover the essential strategies for effectively managing AI-related risks in finance.
Understanding AI risks in finance
Understanding AI risks in finance is essential for organizations looking to innovate while safeguarding their assets. As financial technology evolves, so do the challenges associated with implementing artificial intelligence solutions. Recognition of these risks helps companies prepare effectively.
Types of AI Risks
Various types of AI risks exist in the financial sector. Organizations must be aware of these to navigate potential pitfalls.
- Data privacy issues
- Model bias and fairness
- Regulatory compliance challenges
- Systemic risks from dependencies on AI
Moreover, understanding these risks can lead to enhanced decision-making processes. Financial institutions that embrace a risk management strategy can boost their competitive edge while ensuring compliance. Engaging in open discussions about these risks promotes transparency and trust.
Impact of AI Risks
The impact of AI risks can be far-reaching. For example, poor data usage may lead to inaccurate predictions, impacting financial forecasts. Additionally, biased algorithms can unfairly disadvantage certain groups of customers.
As organizations analyze their use of AI, the goal should be to minimize these risks through rigorous testing and audits. Just as importantly, fostering a culture that prioritizes risk awareness can empower teams to act responsibly.
- Encouraging staff training on AI ethics
- Regular audits of AI systems
- Engagement with regulatory bodies
In summary, understanding the intricacies of AI risks in finance allows organizations to harness technology effectively. By identifying, analyzing, and mitigating these risks, financial institutions can effectively manage uncertainties in their operations, paving the way for innovative yet secure solutions.
Key challenges in AI risk management
In the realm of AI risk management, organizations face several key challenges that can hinder effective implementation. Recognizing these challenges is crucial for devising appropriate strategies to mitigate risks while embracing technological advancements.
Data Quality and Integrity
Maintaining high data quality is vital for successful AI algorithms. Poor data can lead to inaccurate predictions, which amplify financial risks. Companies must consistently evaluate the integrity of their data sources and ensure that data cleansing processes are in place.
- Establishing clear data governance practices
- Regular data audits
- Implementing automated data validation tools
Moreover, algorithms require extensive training data to function optimally. In some cases, organizations struggle to gather diverse and representative datasets. This lack of variety can cause model bias, leading to unfair or incorrect results.
Regulatory Compliance
As AI technology evolves, so does the regulatory landscape. Keeping up with existing regulations and anticipating new ones can be daunting. Organizations must actively monitor compliance requirements to avoid potential penalties.
Furthermore, collaboration with legal and compliance teams is essential. This ensures that AI systems align with industry standards, especially concerning data privacy and security. Involving stakeholders early in the development process can prevent future complications.
- Understanding local and global compliance standards
- Engaging with regulatory bodies for guidance
- Implementing compliance training for staff
Finally, as firms integrate AI systems, they must balance innovation with security. The potential for cybersecurity threats increases with AI’s adoption, making it crucial for organizations to have robust security measures in place.
Both proactive monitoring and continuous improvement of security protocols can significantly reduce vulnerabilities. For this reason, organizations need to foster a culture of security awareness among their employees.
Best practices for implementing AI risk strategies
Implementing AI risk strategies is crucial for organizations looking to navigate the complexities of finance safely. To achieve this, companies can follow some best practices that enhance their ability to manage potential risks effectively.
Establish Clear Objectives
Before implementing any AI solutions, organizations should define clear objectives. This process involves understanding the specific risks they face and how AI can address them. Having well-defined goals not only aligns the team but also aids in measuring success.
- Identify key risk areas
- Set measurable outcomes
- Align AI initiatives with business objectives
Moreover, it is essential to include diverse stakeholders during the planning phase. Engaging various perspectives ensures all potential risks are considered and addressed from the outset, leading to a more robust strategy.
Continuous Training and Development
Another important aspect is investing in continuous training for employees. As AI technology evolves, staff need to stay updated on the latest trends and best practices, which helps them respond effectively to emerging risks.
Regular workshops and training sessions can keep teams informed about technological advancements and regulatory changes. Encouraging a culture of learning enhances the overall risk management framework.
- Implement regular training programs
- Encourage certification in AI and risk management
- Create a knowledge-sharing platform within the organization
In addition, organizations should develop a framework for evaluating the performance of their AI systems. This evaluation helps identify weaknesses in the risk management strategy, allowing for timely adjustments when necessary.
Overall, implementing best practices for AI risk strategies will not only strengthen compliance but also foster innovation. As companies embrace these strategies, they create a safer and more reliable financial environment.
Case studies of AI risk management
Case studies of AI risk management provide valuable insights into how organizations tackle risks associated with artificial intelligence. Analyzing specific examples can help others understand the best practices and strategies that lead to successful risk mitigation.
Financial Institution Example
A well-known bank faced challenges related to algorithmic bias in its lending processes. To address this, the bank implemented a comprehensive risk management strategy that included reviewing its training data for fairness. By doing so, they ensured that the algorithms did not discriminate against certain demographic groups.
Furthermore, the bank conducted regular audits of its AI systems. These audits identified potential biases and allowed the management team to adjust algorithms accordingly, thereby improving fairness in their lending practices.
- Regular bias audits
- Diverse training data
- Feedback loops for algorithm improvements
Healthcare Sector Case
In the healthcare sector, a leading hospital integrated AI to enhance patient diagnosis. However, the hospital faced risks related to patient data privacy. To counter this, they implemented strict data security measures and ensured compliance with regulations like HIPAA.
The hospital trained staff on the importance of data protection and established protocols for handling sensitive information. By prioritizing patient privacy, they minimized risks while benefiting from advanced diagnostic tools.
- Data encryption practices
- Staff training on data privacy
- Regular compliance assessments
These case studies highlight the significance of understanding the specific risks associated with AI implementations. Organizations that analyze such examples can learn from successes and setbacks, refining their own risk management strategies.
Future trends in AI and risk management
Future trends in AI and risk management are set to redefine how organizations approach risks associated with artificial intelligence. As technology continues to advance, businesses must adapt their strategies to stay ahead of emerging challenges and opportunities.
Integration of Machine Learning
One significant trend involves the integration of machine learning algorithms into risk management processes. These algorithms can analyze vast amounts of data quickly, identifying patterns that human analysts may overlook. This capability allows for more accurate risk assessments and proactive strategies.
- Automated risk identification
- Real-time data analysis
- Enhanced predictive analytics
By utilizing machine learning, organizations can not only improve their risk management frameworks but also free up resources for other essential tasks.
Focus on Ethics and Compliance
Another trend is the increasing emphasis on ethical AI usage and compliance with regulations. As businesses deploy AI systems, the potential for ethical dilemmas and compliance issues grows. Organizations will need to adopt frameworks that prioritize ethical considerations while ensuring they meet legal standards.
Training employees on ethical practices will also be crucial. By fostering a culture of accountability, businesses can mitigate risks related to reputation and legal penalties. Examples of measures include:
- Developing clear guidelines for AI use
- Implementing governance structures
- Conducting regular ethical audits
Furthermore, engaging with regulatory bodies proactively will help organizations stay informed about changing standards and ensure compliance.
Embracing Cybersecurity Measures
As AI becomes more prevalent, the risks associated with cybersecurity will intensify. Businesses must adopt robust cybersecurity measures to protect their AI systems and data. This includes regular security assessments, threat monitoring, and incident response strategies to safeguard against cyber attacks.
Investment in cutting-edge security technologies will also play a key role. Companies that prioritize cybersecurity will be better equipped to manage risks while leveraging AI’s potential to enhance their operations.
In conclusion, understanding the landscape of AI risk management is essential for organizations today. By embracing best practices, learning from case studies, and keeping up with future trends, businesses can effectively navigate the complexities of implementing AI technologies. As AI continues to evolve, focusing on ethical use, compliance, and security will help mitigate risks and unlock new opportunities for growth.
FAQ – Frequently Asked Questions about AI Risk Management
What are the key challenges in AI risk management?
Key challenges include data quality, algorithmic bias, regulatory compliance, and cybersecurity threats.
How can organizations ensure ethical AI use?
Organizations can ensure ethical use by developing clear guidelines, providing training, and conducting regular audits.
Why are case studies important for AI risk management?
Case studies provide real-life examples that help organizations learn from successes and mistakes in implementing AI solutions.
What future trends should companies watch in AI and risk management?
Companies should watch trends like machine learning integration, ethical considerations, and advancements in cybersecurity measures.